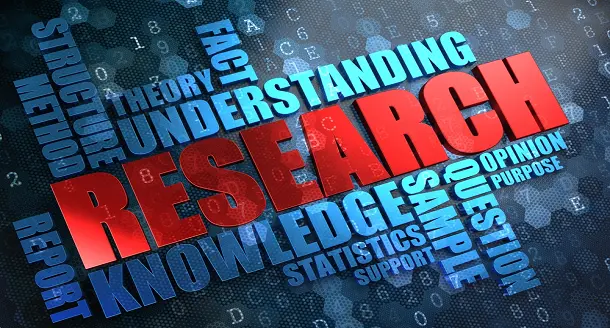
To answer the question, “What are the kinds of variables and their uses?” you must first understand each of them. Variables are classified into intervening, Dependent, and Composite variables. Each type has its unique characteristics and uses. Understanding these differences will help you select the correct variables for your study.
Intervening variables:
Intervening variables explain a causal relationship between two variables in a study. They are not real variables, but they provide a mediation effect that helps us to understand experimental results. An example would be a correlation between education and reading ability. The education variable, then, can explain this association.
Using intervening variables is crucial in studies that aim to predict outcomes. They help us understand the causes of diseases and the effect of interventions. They are also critical in establishing causal chains in theoretical models. You can use several methods to test for the presence of an intervening variable.
Dependent variables:
A study may depend on Dependent and independent variables to determine a specific result. For instance, a study about depression may find that the type of therapy used helps patients with depression. An experimenter will modify an independent variable to see if it affects the dependent variable. For example, the type of information given to a group of participants could influence the time participants spent remembering the information.
Another example of a study that uses dependent and independent variables is when a student’s graduating class depends on the school’s CGPA, grade, and rating scale. A person’s salary may also be dependent on these factors. As a result, the amount of income someone makes can impact their quality of life.
Composite variables:
Composite variables are useful for altering the strength of relationships between variables in a study, but these can also be difficult to interpret and reduce statistical power. This presentation introduces the methods for creating composite variables and shows how they are used in actual studies. The exhibition illustrates some common types of composite variables and how they can enhance the quality of statistical analyses.
When using composite variables, remember to keep the components of the variables comparable to ensure that they are interchangeable. They should have similar clinical importance and sensitivity to treatment. A good example is a composite health score that includes blood pressure, weight, and chronic pain.
Nominal variables:
The first step in analyzing nominal data is to define the purpose of collecting the data. This will enable you to develop guiding questions, test your hypothesis, and choose the appropriate measurement methods. Nominal data can be presented in various ways, such as pie charts and bar graphs.
Nominal variables are the most basic type of variables. They can take either a numeric or a qualitative value. A common example is the gender of a person. These types of variables are often used in social science research.
Distinctive variables:
Variables are numbers that have a specific value or range. Examples of these types of variables include age, weight, grades, and the province of birth. There are also ordinal and categorical variables. These two types of variables have different uses. Each has its advantages and disadvantages and another kind of measurement.
A function has two different derivatives, one for each variable. The first is the default differentiation variable, and the second is the second derivative.
Numeric variables:
A numerical variable is a measurable quantity that has a specific numerical value. A numerical variable can be either continuous or discrete. For example, a variable can be height, weight, or blood pressure. Its value can be as large or small as its measurement instrument allows.
To analyze and report numerical data, you must understand the properties of these variables. You can use measures of central tendency and dispersion to understand the properties of these variables. Knowing these properties will help you interpret the results of a study in a more understandable way.
Continuous variables:
Continuous variables are numerical expressions whose value varies. They can take any numeric value and are generally measured on a scale. In statistical analysis, they are used in statistical models. They have many uses in data analysis. In addition to data collection, they are useful in modelling processes, such as machine learning.
Continuous variables are more difficult to represent visually than discrete ones. Using bar charts, for example, highlights the distinct nature of each value. On the other hand, discrete variables are based on counting data points.
Author Bio:
Carmen Troy is a research-based content writer, who works for Cognizantt, a globally recognized professional SEO service and Research Prospect; an 论文和论文写作服务 Mr Carmen holds a PhD degree in mass communication. He loves to express his views on various issues, including education, technology, and more.