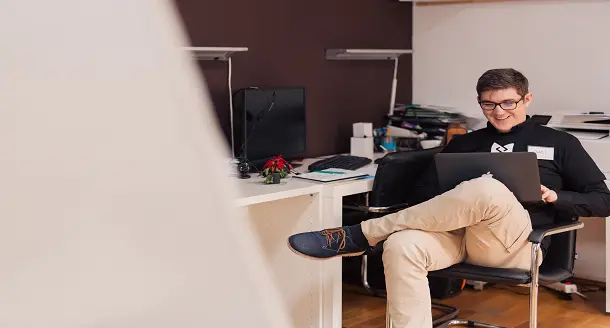
There are many Sampling Methods used to collect data. This article will discuss several of them, including Multistage, Cluster, and Experience sampling. The main goal of these methods is to reach a good spread of the target population. This can be achieved by specifying quotas, such as a specific proportion of males and females or age or ethnic groups. However, it is important to consider that bias can enter the selection process.
Experience sampling:
Experience Sampling Methods is a technique in which researchers collect data from people through a diary. This method has many applications, including teletherapy and research. It is important to note that today’s techniques and tools are more advanced than those used decades ago. For example, new software tools make the process easier to complete.
Experience sampling is a technique that involves gathering data from people in real life by letting them self-report their feelings, symptoms, and environment. This method’s key is collecting data at the right moment and situation. For example, participants might be paged several times to record specific aspects of their day.
Stratified Sampling Methods:
Stratified sampling is a probability-based approach to sampling a population of interest. It aims to create a proportionally balanced sample from a target population. It involves defining the criteria for recruitment, including quotas for certain demographic groups such as males and females, specific age groups, and ethnic groups. Stratified sampling is useful for studies involving heterogeneous populations, but it is not always possible to eliminate bias from the selection process.
The number of strata in a sample depends on the study’s goals. For example, strata can be defined by gender to study a subgroup, or they may be determined by age or education level. Strata should be identifiable.
Cluster sampling:
Cluster sampling is a time and cost-efficient sampling method example, especially for surveys of large populations. It utilises a clustered sample design with randomisation. The sampled clusters will have high external validity because they will reflect the characteristics of the larger population. However, the internal fact of the sampled classes is lower, especially if there are more stages of clustering. This type of sampling also requires more planning than other sampling methods.
The key to implementing cluster sampling is identifying the fine lines between subgroups. The clustering parameters must be set so that groups are internally similar, ye diverse. This ensures that relevant data will be gathered from a broad range of populations.
Multistage Sampling Methods:
Multistage sampling is a method used in large populations to gather data. This method divides the population into smaller groups using hierarchical classifications, making it feasible to obtain a representative sample. The advantage of this method is that the results can be made more reliable because of all the elm population elements represented in the sample model. This method has drawbacks; it is flexible and cost-effective, especially for large samples. It is also more flexible than a simple random sample.
Random sampling:
Random sampling is a Sampling Method that allows for a wide range of results. Its advantages include the ability to calculate the error of sampling and the ability to reduce selection bias. Moreover, it is one of the most straightforward methods for probability sampling. However, it is not without limitations. For example, choosing enough individuals with the characteristics and interests may be difficult. Furthermore, it can be challenging to contact individuals differently.
One of the challenges with this method is the possibility of bias in the results. A biased sample can be used to support a particular viewpoint. For example, one group may try to demonstrate their support for candy in the classroom by surveying students right before lunch. Another technique is called cherry picking, which consists of only choosing individuals who support the viewpoint of the researchers.
Author Bio:
Owen Ingram is a research-based content writer who works for Cognizantt, a globally recognized E-Commerce-SEO and Research Prospect; a Dissertatie schrijven diensten tegen de beste prijzen in het Verenigd Koninkrijk Mr Owen Ingram holds a PhD degree in mass communication. He loves to express his views on various issues, including education, technology, and more.